Artificial Intelligence in Medical Devices 101
- meganjungers
- Feb 10
- 7 min read
Artificial intelligence is undoubtedly one of the most promising elements in the future directions of healthcare. With a more tailored and personalizable approach to medicine, AI in the healthcare setting is contributing to an industry-wide trend toward patient-centered care1. Further, the excitement for pairing artificial intelligence with medical device technology has sparked innovation across the globe at unprecedented rates. For reference, by the end of 2024, the FDA had authorized over 1,000 AI-enabled devices for pre-approval for healthcare use`1.
However, there is significant regulatory divergence across each governing nation. Some policies are merely relaying standards of best practice, while others are strongly enforcing regulations on what data can and cannot be used for AI purposes. The differences in these "hard" versus "soft" attitudes toward policy both hold ethical considerations that we will continue to discuss, but it can be challenging to understand which policies apply to respective uses of AI.
On this platform, we will be focusing on a small niche of AI-enabled medical devices- ones that modulate neuronal functions. These are called aDBS devices, and we will include a more in-depth analysis of aDBS here. However we first must learn about the different ways that AI functions so that we can best interpret each AI policy correctly with how it pertains to our specific technology of interest.
What is Artificial Intelligence?
We start by working to understand the fundamental basis of AI and what type of AI is included in aDBS.
"Artificial intelligence" refers to the different types of predictive language models that mimic, predict, and produce outputs comparable to human intelligence2. There are however different subsets of AI based on how information is learned3. The most notorious of those subset types is machine learning (ML), which is a continuously adapting model of artificial intelligence that can develop its own rules from input data that is trained and improved through multiple series' of data processing3. This occurs through different feedback mechanisms3:
supervised learning - human-driven training using pre-labeled or historical data from a human expert/ trainer
predict outcomes based on classifications/regressions
unsupervised learning - data-driven results based the sample given
predict outcomes and build subgroups based on data-clustering
reinforcement learning - environmental-driven with interactive learning cycles
predict outcomes based on results from reinforcement training towards a pre-defined target (series of rewards and penalties teach model correct outcomes)
Machine learning further has an array of different algorithm subcategories, but the one of interest with aDBS is called deep learning (DL). Deep learning includes applying a neural network model of multiple hidden processing layers consisting of combinations of supervised, unsupervised, and reinforcement learning ML approaches to mimic sequence processing in the human brain3. The immense potential associated with these neural network models in healthcare has sparked innovation across the field, and AI is finding opportunities to support clinicians in a host of tasks in their jobs. These range from disease monitoring to radiology and diagnostic tools to predictive outcome modeling and even treatment/therapeutic options2,3. The complexities of deep learning are much higher than other basic AI models, and accountability for outputs and decisions of these algorithms can be challenging given the multitude of layers involved at the coding level.
Due to their intricate design, there are at least three layers of processing in neural networks, but when models that have multiple items being assessed (such as brain wave frequencies of hundreds of thousands of neurons being computed in a localized area of the brain) there can be as many as 10 layers for one singular output, making it difficult to follow all reasoning for every part of an algorithm4,5. For design purposes, this contributes to one of the largest fears relating to trust in AI, which is sometimes referred to as the "black-box" question (when ML engineers cannot explain how an output is produced from a neural network)5. It additionally requires much more data to be accurately trained on, making the role of sourcing data a huge component of the development process5.
So, when we are referring to AI policy concerning aDBS devices, we are trying to identify how it applies to a very small class (neural networks) of the different types of AI under a specific regulation or guidance. This can be difficult to interpret given the different ways these technical terms are used or the indirect application under broad legislation, among other challenges.
The current state of artificial intelligence in medicine is also considered to only be in the early stages of its potential capabilities. The present level of development for AI is considered to only be limited to applications as it relates to programmed tasks, called artificial narrow intelligence3. AI is predicted to have at least two subsequent phases of development, artificial general intelligence, where AI will be able to reason, argue, and problem-solve like people, and then artificial superintelligence, where AI will have cognitive capacities that are greater than that of a human3. Given the likelihood that AI will progress to these stages, creating clear policy as it applies to respective AI elements can ensure that accountability and trustworthiness are maintained in crafting future AI.
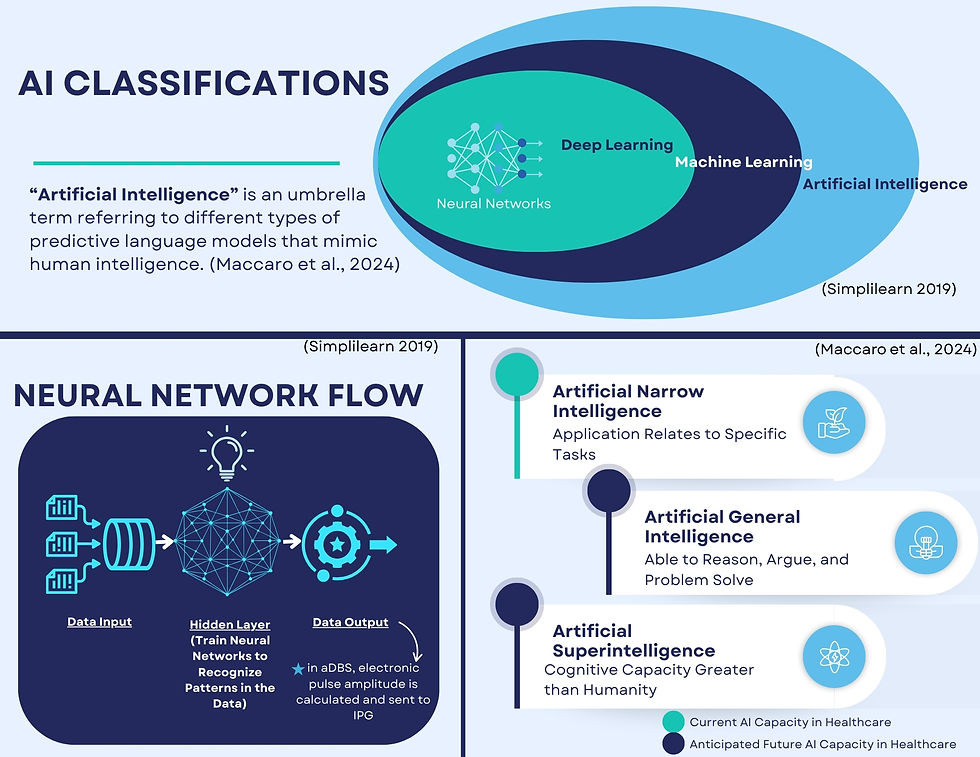
Closed Loop vs. Open Loop
Lastly, we will touch on where AI is utilized in aDBS technology, and take a high-level look at why it improves upon current DBS technology. Again, for an in-depth look into DBS, visit this link.
Standard DBS utilizes an implanted pulse generator (IPG) to send specific electric signals to targeted neurons in the brain through an implanted lead. The technology has not evolved much since its first uses for treating Parkinson's Disease in the 1990s, and while the device significantly improves the quality of life for many patients using this treatment option, DBS is programmed to a fixed setting that entirely may not account for all variances needed in managing neuronal activity throughout the day4. This is called an open-loop circuit, as the flow of data terminates in an output, which is never reassessed6.
The incorporation of AI into DBS allows for neuro-feedback based on each individual patient in any given context4, The goal of aDBS is to shorten time delays in stimulation changes to be rather seamless reactions, ultimately managing brain signaling abnormality variances in real-time4, The neural network is designed to assess the recording of brain activity, decode the current state of the brain, and output the necessary stimulation frequency to return the brain to a more normalized state4. This is called a closed-loop circuit, as the feedback of data is continuous and helps the device improve and adapt its outputs in real time6.

While this is a rather small component of the device, aDBS still does include AI in its design, subjecting it to respective privacy, medical device, and AI regulations and policies. There is also the concern of how other brain computer interface (BCI) systems will be subject to these policies as their programming capabilities become more advanced. aDBS technology currently has the capacity to only measure neural activity, but should BCIs develop the ability to interpret activity for cognitive processes, there are worries of infringement on mental autonomy, data privacy, and even the potential of the device itself evolving a form of system autonomy6. Such issues about the future of AI can only be possibly mitigated by responsible guidances, however the current international regulatory landscape leaves some gaps for potential AI misconduct.
Moreover, there is the pressing concern of what role data plays in AI, and how regulations will filter or allow what data is available to shape AI technology. Al algorithms are the result of the data they are trained on, and biases are reflected in that respective data6. Additionally, if the programmer and engineer possesses biases from their learned experiences, those will be reflected in the data outputs2.
In healthcare, the risks associated with not addressing AI bias are very high for patients. For one, the underrepresentation of diverse populations (ethnic, socioeconomic, and demographic groups) in data can lead to inaccurate outputs; in the context of healthcare, this can have incredibly dangerous repercussions for patient safety with regard to their diagnoses and treatment2. A patient further might not understand how biases in AI could ultimately impact their care, and how that would differ from only the biases of their provider2. AI only stands to further complicate the issue of informed consent, which can lead to patients misunderstanding what they are consenting to regarding their care or disclosure of data. Therefore, if a programmer has only experience working with one or two demographics, their understanding of medicine and patient care will be directly reflected in the outputs of the machine learning model7. The dangers of not addressing these elements of programmer bias could potentially reflect larger historical and societal inequities in healthcare7.
Ultimately, intentional AI policy can help guide development for medical devices so as to best address these concerns of the potential for misconduct, prioritizing patient safety, and managing the influence of bias in AI.
Citations:
U.S. Food and Drug Administration. 2025. "FDA Issues Comprehensive Draft Guidance for Developers of Artificial Intelligence-Enabled Medical Devices." FDA News Release, January 6, 2025. https://www.fda.gov/news-events/press-announcements/fda-issues-comprehensive-draft-guidance-developers-artificial-intelligence-enabled-medical-devices.
Maccaro, Alessia, Katy Stokes, Laura Statham, Lucas He, Arthur Williams, Leandro Pecchia, and Davide Piaggio. 2024. "Clearing the Fog: A Scoping Literature Review on the Ethical Issues Surrounding Artificial Intelligence-Based Medical Devices." Article in Journal of Personalized Medicine 14 (5): 443." https://www.mdpi.com/2075-4426/14/5/443
Christos, and Konstantinos Perakis. 2023. "Artificial Intelligence, Machine Learning and Deep Learning." Clinical Microbiology and Infection. https://www.journalofinfection.com/article/S0163-4453(23)00379-1/fulltext
Arlotti, Mattia, Matteo Colombo, Andrea Bonfanti, Tomasz Mandat, Michele Maria Lanotte, Elena Pirola, Linda Borellini, et al. 2021. "A New Implantable Closed-Loop Clinical Neural Interface: First Application in Parkinson's Disease." Frontiers in Neuroscience 15: 763235. https://pmc.ncbi.nlm.nih.gov/articles/PMC8689059/
Rodriguez, Fernando, Shenghong He, and Huiling Tan. 2023. "The Potential of Convolutional Neural Networks for Identifying Neural States Based on Electrophysiological Signals: Experiments on Synthetic and Real Patient Data." Frontiers in Human Neuroscience 17: 1134599. https://pmc.ncbi.nlm.nih.gov/articles/PMC10272439/.
Haeusermann, Tobias, Cailin R. Lechner, Kristina Celeste Fong, et al. 2021. "Closed-Loop Neuromodulation and Self-Perception in Clinical Treatment of Refractory Epilepsy." AJOB Neuroscience 12 (3): 187–200. Figure 1. https://pubmed.ncbi.nlm.nih.gov/34473932/
James, Ted A. 2024. "Confronting the Mirror: Reflecting on Our Biases Through AI in Health Care." Harvard Medical School Postgraduate Education, September 24, 2024. https://postgraduateeducation.hms.harvard.edu/trends-medicine/confronting-mirror-reflecting-our-biases-through-ai-health-care#:~:text=The%20bias%20in%20AI%20outputs,uneven%20distribution%20of%20medical%20resources.
Comments